AI Algorithms In Autonomous Vehicles
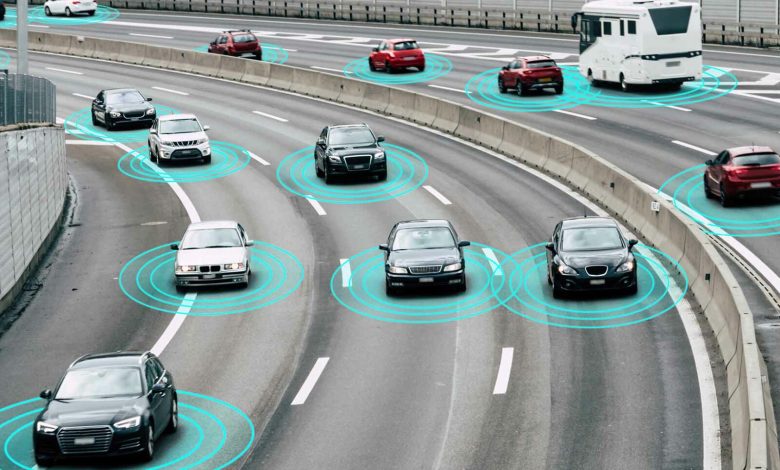
The transportation industry is undergoing a radical change thanks to artificial intelligence (AI), as autonomous(self-driving) cars with sophisticated electronics are now able to navigate and make judgments in real time while on the road. Autonomous cars that use artificial intelligence (AI) promise safer and more effective ways of transportation, which might eventually reduce the number of fatalities brought on by human error.
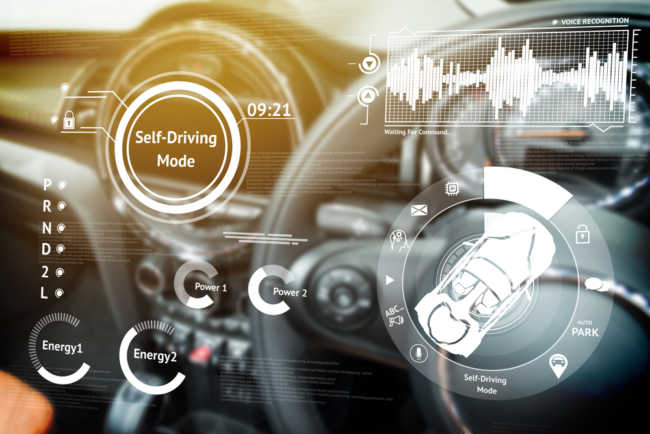
Sensing the Surroundings: Perception Algorithms
Algorithms that can learn from data and get better without explicit programming are used in machine learning in autonomous cars. To see and comprehend their environment, the cars gather and process data from a variety of sensors, including cameras, lidar, radar, and GPS. After that, machine learning models are fed this data in order to identify relevant trends, forecast the future, and provide driving behaviors that are suitable. Over time, the autonomous cars can improve their driving skills thanks to machine learning algorithms that go through an iterative process of training and improvement. https://enoughinfo.com
Autonomous vehicles are equipped with an array of sensors, including cameras, LiDAR, radar, and ultrasonic sensors, to perceive and interpret their surroundings. Perception algorithms, powered by deep learning and computer vision techniques, analyze sensor data to identify objects such as pedestrians, vehicles, road signs, and obstacles. These algorithms enable the vehicle to create a real-time, high-definition map of its environment, crucial for navigation and decision-making.
Making Sense of Data: Sensor Fusion and Localization
To navigate safely, autonomous vehicles must accurately localize themselves within their environment and track the movement of surrounding objects. Sensor fusion algorithms integrate data from multiple sensors to create a comprehensive understanding of the vehicle’s position and orientation relative to its surroundings. By combining information from GPS, inertial measurement units (IMUs), and other sensors, these algorithms provide robust localization capabilities, even in challenging conditions such as urban canyons or adverse weather.
Decision-Making in Action: Planning and Control Algorithms
Once the vehicle has perceived its environment and determined its position, it must make intelligent decisions to navigate from point A to point B safely and efficiently. Planning algorithms generate a trajectory or series of actions that optimize factors such as safety, comfort, and adherence to traffic laws. Utilizing techniques from motion planning and reinforcement learning, these algorithms account for dynamic obstacles, traffic conditions, and route preferences to determine the optimal path for the vehicle.
Control algorithms, on the other hand, translate the planned trajectory into precise commands for the vehicle’s actuators, including steering, acceleration, and braking. By continuously adjusting these control inputs based on real-time sensor feedback, the vehicle can execute smooth, responsive maneuvers while maintaining stability and adherence to safety constraints.
Learning and Adaptation: Machine Learning in Autonomous Vehicles
One of the most compelling aspects of autonomous vehicles is their ability to learn and adapt to new environments and scenarios. Machine learning algorithms, including neural networks and reinforcement learning, enable vehicles to improve their performance over time through experience and data-driven feedback. Whether it’s refining perception capabilities, optimizing driving behaviors, or predicting the intentions of other road users, machine learning empowers autonomous vehicles to continually enhance their decision-making prowess.
Overcoming Challenges: Safety, Reliability, and Regulation
While the promise of autonomous vehicles is immense, significant challenges remain to be addressed. Ensuring the safety and reliability of AI algorithms in real-world driving scenarios is paramount, requiring rigorous testing, validation, and verification processes. Moreover, ethical considerations surrounding issues such as algorithmic bias, transparency, and accountability must be carefully navigated to foster trust and acceptance among stakeholders and the public.
Regulatory frameworks and standards play a crucial role in shaping the deployment and adoption of autonomous vehicles, balancing innovation with safety and societal concerns. Collaborative efforts between industry, academia, and government are essential to establish guidelines and best practices that foster innovation while safeguarding public welfare.
Looking Ahead: Towards a Connected and Autonomous Future
Additional Autonomous Driving Learning Algorithms: Deep learning algorithms such as Recurrent Neural Networks (RNNs) and Convolutional Neural Networks (CNNs) are frequently employed. While RNNs evaluate sequential data to forecast future events and trajectories, CNNs use visual data for object detection.
As AI algorithms continue to evolve and mature, the vision of fully autonomous vehicles seamlessly navigating our roads inches closer to reality. Beyond individual vehicles, the integration of autonomous technology with smart infrastructure and vehicle-to-everything (V2X) communication promises to further enhance safety, efficiency, and sustainability in transportation systems.
In summary, artificial intelligence and machine learning are transforming autonomous driving, improving its safety and effectiveness. We may anticipate even more complex AI algorithms as technology develops.
From reducing traffic congestion and emissions to improving accessibility and mobility for all, the potential benefits of autonomous vehicles driven by AI algorithms are vast and transformative. As we embark on this journey towards a connected and autonomous future, collaboration, innovation, and a steadfast commitment to safety will be paramount in realizing the full potential of this groundbreaking technology.