How To Apply AI for Predictive Maintenance in Manufacturing
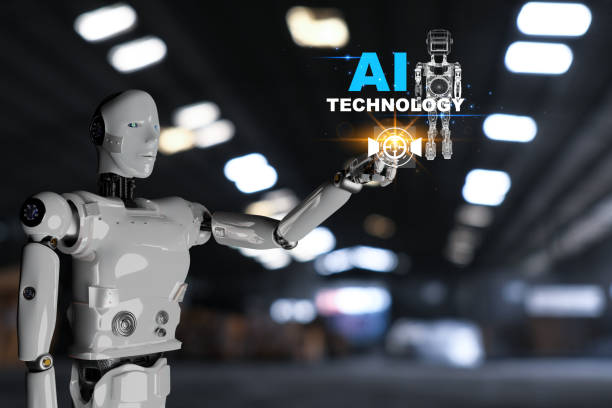
Imagine a world where machines can predict when they’re going to break down. A world where manufacturers can avoid costly downtime and keep their production lines running smoothly.
This is the world of AI-powered predictive maintenance.
AI-powered predictive maintenance uses artificial intelligence to analyze data from machines and sensors to identify patterns and trends that can predict when a machine is likely to fail.
This information can then be used to schedule maintenance and repairs before they’re needed, preventing downtime and costly equipment failures.
Benefits of AI-powered predictive maintenance
There are many benefits to using AI-powered predictive maintenance in manufacturing, including:
- Reduced downtime: AI-powered predictive maintenance can help manufacturers to reduce downtime by identifying and fixing potential problems before they cause a machine to break down.
- Increased productivity: By reducing downtime, AI-powered predictive maintenance can help manufacturers to increase productivity.
- Reduced costs: AI-powered predictive maintenance can help manufacturers to reduce costs by avoiding costly equipment failures and downtime.
- Improved safety: AI-powered predictive maintenance can help manufacturers to improve safety by identifying potential hazards before they cause accidents.
How to apply AI for predictive maintenance in manufacturing
To apply AI for predictive maintenance in manufacturing, you will need to:
- Collect data: The first step is to collect data from your machines and sensors. This data can include things like vibration, temperature, and performance metrics.
- Choose an AI platform: There are a number of different AI platforms available that can be used for predictive maintenance. Choose a platform that is right for your needs and budget.
- Train the AI model: Once you have chosen an AI platform, you will need to train the AI model on your data. Ptc.com says this will allow the model to learn to identify patterns and trends that can predict when a machine is likely to fail.
- Deploy the AI model: Once the AI model is trained, you can deploy it to your production environment. This will allow the model to monitor your machines and send alerts when potential problems are detected.
Tips for successful AI-powered predictive maintenance
Here are a few tips for successful AI-powered predictive maintenance:
- Use the right data: The quality of your data is essential for the success of your AI-powered predictive maintenance program. Make sure to collect high-quality data from your machines and sensors.
- Choose the right AI platform: There are a number of different AI platforms available that can be used for predictive maintenance. Choose a platform that is right for your needs and budget.
- Train the AI model properly: The AI model needs to be trained on a large and diverse dataset in order to be effective. Make sure to train the model on data from a variety of machines and operating conditions.
- Monitor the AI model: Once the AI model is deployed, you need to monitor its performance and make sure that it is still accurate and effective.
- Take action on alerts: When the AI model sends an alert, it is important to take action immediately to prevent a machine failure.
FAQs about How to apply AI for predictive maintenance in manufacturing
What are the different types of AI-powered predictive maintenance models?
There are two main types of AI-powered predictive maintenance models:
- Machine learning models: Machine learning models are trained on historical data to learn to identify patterns and trends that can predict when a machine is likely to fail.
- Deep learning models: Deep learning models are a type of machine learning model that uses artificial neural networks to learn from data. According to linkedin, deep learning models are often more accurate than machine learning models, but they require more data to train.
What are some of the challenges of implementing AI-powered predictive maintenance?
Some of the challenges of implementing AI-powered predictive maintenance include:
- Cost: AI-powered predictive maintenance solutions can be expensive, especially for small and medium-sized businesses.
- Data requirements: AI-powered predictive maintenance models require a large amount of data to train. This data can be difficult and expensive to collect, especially for small and medium-sized businesses.
- Complexity: AI-powered predictive maintenance systems can be complex to implement and maintain. This may require specialized skills and expertise.
How can I overcome the challenges of implementing AI-powered predictive maintenance?
Here are a few tips for overcoming the challenges of implementing AI-powered predictive maintenance:
- Start small: Don’t try to implement a complex AI-powered predictive maintenance solution all at once. Start with a small and manageable project.
- Use a cloud-based solution: Cloud-based AI-powered predictive maintenance solutions can help to reduce the cost and complexity of implementation.
- Work with a partner: If you don’t have the in-house expertise to implement and maintain an AI-powered predictive maintenance system, you may want to work with a partner who can help you.
What are some of the best practices for implementing AI-powered predictive maintenance?
Here are some best practices for implementing AI-powered predictive maintenance:
- Define your goals: What do you want to achieve with AI-powered predictive maintenance? Do you want to reduce downtime, increase productivity, or reduce costs? Once you know your goals, you can choose the right AI-powered predictive maintenance solution and metrics to track.
- Collect the right data: The quality and quantity of your data is essential for the success of your AI-powered predictive maintenance program. Make sure to collect high-quality data from your machines and sensors.
- Choose the right AI platform: There are a number of different AI platforms available that can be used for predictive maintenance. Choose a platform that is right for your needs and budget.
- Train the AI model properly: The AI model needs to be trained on a large and diverse dataset in order to be effective. Make sure to train the model on data from a variety of machines and operating conditions.
- Monitor the AI model: Once the AI model is deployed, you need to monitor its performance and make sure that it is still accurate and effective.
- Take action on alerts: When the AI model sends an alert, it is important to take action immediately to prevent a machine failure.
Conclusion
AI-powered predictive maintenance is a powerful tool that can help manufacturers to reduce downtime, increase productivity, reduce costs, and improve safety.
By following the best practices above, you can successfully implement AI-powered predictive maintenance in your manufacturing operation.
I believe that AI-powered predictive maintenance is essential for manufacturers in the 21st century. By using AI to predict when machines are likely to fail, manufacturers can avoid costly downtime and keep their production lines running smoothly.
I encourage all manufacturers to invest in AI-powered predictive maintenance. It is one of the best investments you can make to improve the efficiency and profitability of your manufacturing operation.
Additional tip
Don’t be afraid to experiment: AI-powered predictive maintenance is a new technology, so there is no right or wrong way to do it. Experiment with different approaches and solutions to find what works best for your business.